AI in Finance: Opportunities, Sustainability, Ethics
By Angelique Nivet
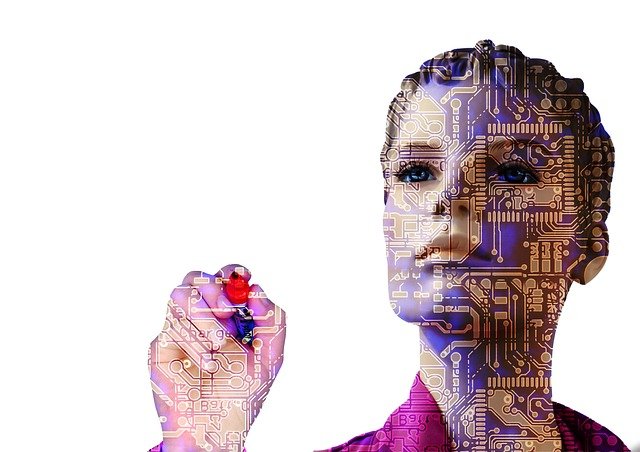
AI: Financial and Environmental Impacts
The Rise of AI in Finance
Artificial intelligence (AI) generally refers to the ability of machines to exhibit human-like intelligence. More precisely, AI can be defined as a suite of technologies and capabilities enabled by adaptive predictive power and exhibiting some degree of autonomous learning. When adopted, AI can substantially increase the ability of firms to recognize patterns, anticipate future events and make better informed decisions (Deloitte, “Artificial intelligence in investment management”).
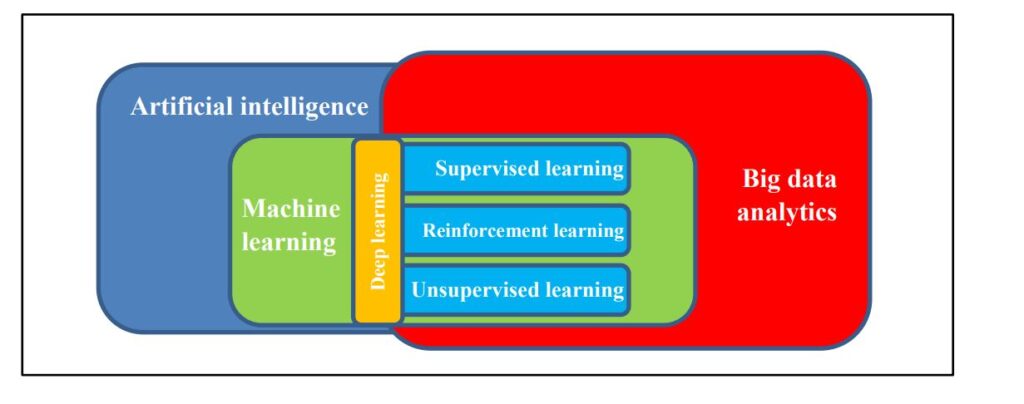
Today’s rapid data proliferation coupled with the exponential rise in computing power have accelerated AI’s prominence in the financial sector enhancing the accuracy of business predictions while enabling a deeper understanding of key economic drivers. As businesses across the globe increasingly face regulatory, reputational, and market-driven pressure to adapt and embrace the shift to a low-carbon sustainable future, the adoption of AI in finance offers a challenging and deep transformative pathway for innovation. It is thus critical for financial players, business leaders and technology providers alike to think ahead about the economic, societal, and environmental benefits, as well as the risks created by AI.
Responsible AI and Sustainability
AI offers groundbreaking possibilities for economic growth and CO2 emissions reduction as digitalization and decarbonization take hold globally. According to a 2020 study by PwC UK, using AI for environmental applications has the potential to boost global GDP by 3.1 – 4.4% while also reducing global greenhouse gas (GHG) emissions by around 1.5 – 4.0% by 2030, compared to business as usual (Gillham et al., “How AI can enable a sustainable future”).
The application of AI across four key sectors – energy, transport, water, and agriculture – has the potential to generate a gain of US$3.6 – $5.2 trillion mainly driven by an optimized use of inputs, a higher output productivity and the automation of routine manual tasks (Gillham et al., “How AI can enable a sustainable future”). Using AI in these four sectors can accelerate the move to a low-carbon economy with a reduction in worldwide GHG emissions equivalent to the predicted 2030 annual emissions of Australia, Canada and Japan all combined.
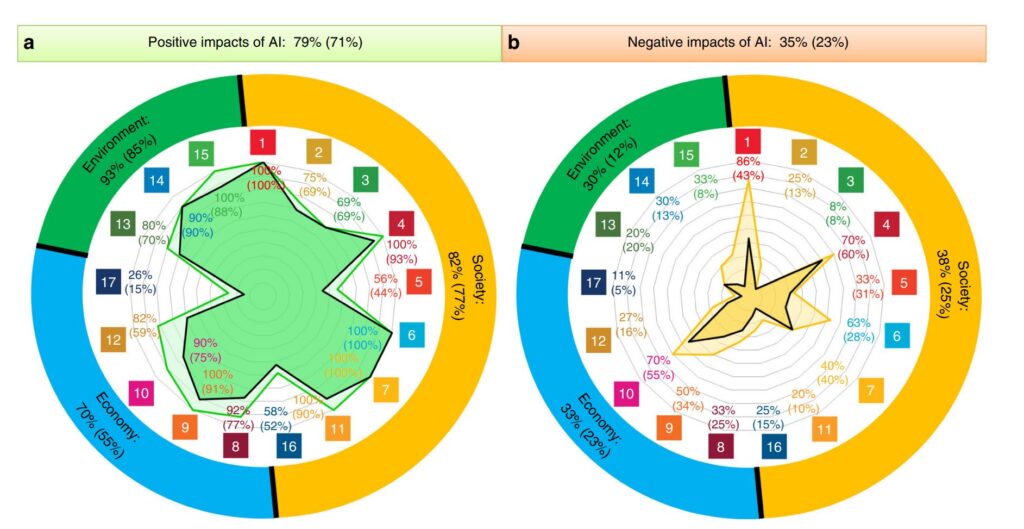
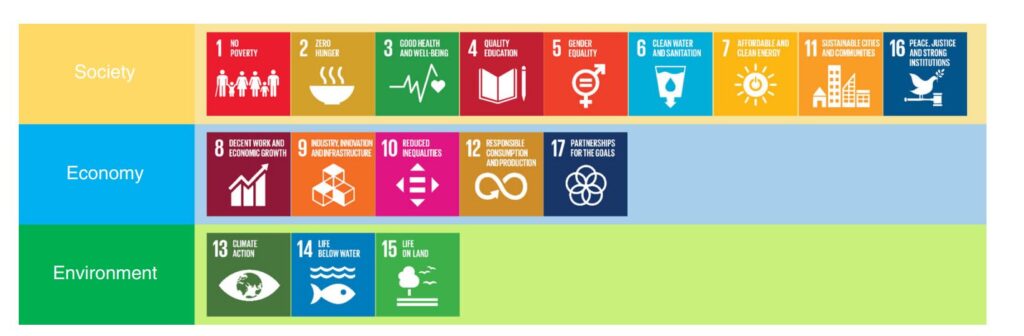
Responsible AI has the potential to act as a powerful tool that helps companies rethink how algorithms are built, deployed, maintained, and used while enabling wealth managers to provide better-informed investment advice. Responsible AI also helps direct investment away from non-sustainable industries and assets (The Fintech Times, “DreamQuark: The Sustainability Imperative for Financial Services to Embrace Responsible AI). Responsible AI and sustainable digital finance are thus integral to the future of the financial and technological industries. In managing the sustainable transition successfully, financial and technology players alike share the responsibility of building an environmentally conscious AI market.
AI allows investors to collect and process unparalleled amounts of data while accounting for Environmental, Social and Governance (ESG) risks and opportunities. Much of the potential for AI in ESG investing importantly stems from sentiment analysis algorithms. Sentiment analysis programs are trained to read conversation types and analyze tone by comparing the words used to a reference set of existing information. A program trained to read the transcripts of a company’s quarterly earnings calls could, for example, use natural language processing to identify parts of the conversation in which a CEO makes ESG-related comments and then infer from the words used the company’s commitment to climate change mitigation (S&P Global, “How can AI help ESG investing?”).
Sustainable AI in finance consequentially calls for enhanced collaboration between financial institutions and regulatory authorities. The increase of technology-specific regulation and guidance is key to foster durable commitment to AI technology in finance. Clearer communication of regulatory expectations and the facilitation of audit processes are in particular essential to the widespread adoption of sustainable AI. Macro-level standards, coordinated regulation and sustained international links, in addition, are necessary for the implementation of responsible AI usage across different countries and jurisdictions (Williams et al., “Sustainable AI in Finance: Understanding the Promises & Perils”).
AI in Energy, Transport, Water and Agriculture
Beyond the reduction of GHG emissions, AI offers a wide range of environmental and financial benefits. Such benefits notably relate to the preservation of water quality and biodiversity and the reduction of air pollution and deforestation. According to a 2020 study conducted by PwC UK, the use of AI has the largest impact on GHG emissions reduction in the energy and transport sectors. Although AI’s impact on the sectors of agriculture and water were found to be smaller, AI technology has a significant effect on the protection of natural resources, fauna and flora (Gillham et al., “How AI can enable a Sustainable Future”).
In the energy sector, core features of AI’s economic and environmental benefits include smarter monitoring and management of energy consumption, optimized energy use through the automation of price responsiveness to market signals and better forecasting of short- and long-term energy needs. AI can further be used to enhance the operational efficiency of fossil fuel assets and boost the energy production of renewable assets. As concerns the transport sector, in turn, AI tools can be used to produce autonomous vehicles as well as foster eco-driving features, traffic flow optimization and smart pricing for vehicle tolls. AI technology is also useful to predict maintenance needs for vehicles which presents both economic and logistical benefits.
In the water sector, AI enables the intelligent tracking of water use at industrial and household levels to predict demand and reduce both wastages and shortages. In addition, AI is a helpful tool to model water treatment processes and foster the recycling of greywater. It is important to note that predicting and monitoring water demand can reduce the water sector’s electricity consumption and consequently, CO2 emissions. Lastly, AI can be used to map agricultural activities to promote better farm management and a stronger enforcement of regulation. Using AI robotics to monitor agricultural conditions can for example help prevent the spread of diseases among livestock and thus avoid critical financial losses for farmers.
AI in Investment Management, Banking and Fighting FinCrime
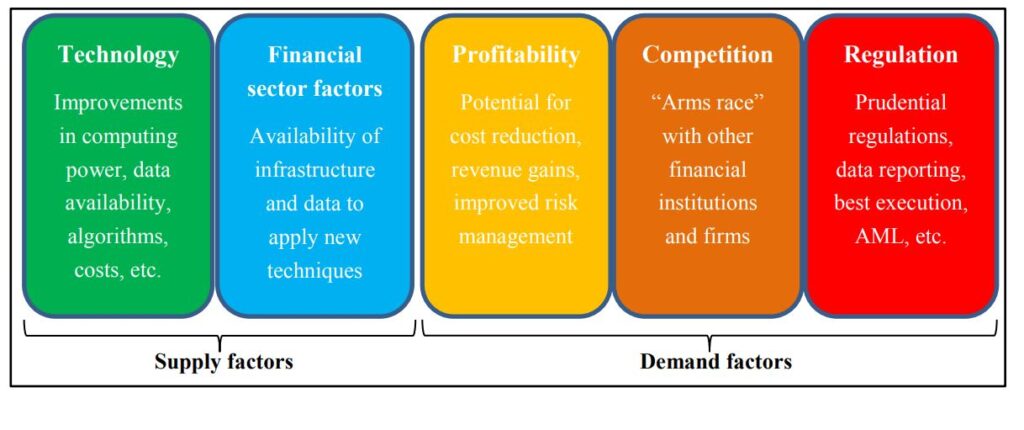
AI in Investment Management
By using AI, investment management firms are empowered to reshape operating models, augment the intelligence of the human workforce and facilitate the development of next-generation, cutting-edge capabilities (Deloitte, “Artificial intelligence in investment management”). AI is also relevant for analyzing alternative datasets such as weather forecasts, monitoring suspicious transactions and triggering response protocols, as well as generating reports for clients, portfolio commentary and risk commentary.
The new opportunities that AI brings to the financial investment sector extend far beyond cost reduction and efficient operations. Many investment management firms have already taken note of AI’s potential benefits and are actively applying cognitive technologies to various business functions across the industry value chain. BlackRock, the world’s largest asset manager, has recently set up a new center dedicated to research in AI, the “BlackRock Lab for Artificial Intelligence”, illustrating the rising interest in AI among leading firms (Deloitte, “Artificial intelligence in investment management”).
In its 2019 report on the use of AI in investment management, Deloitte underlines four key transformational abilities of AI:
- Generating Alpha and boosting organic growth.
- Heightening operational efficiency.
- Generating a more comprehensive understanding of investor preferences.
- Managing risks more effectively while economizing resources.
AI in Banking
Amid the current Fintech revolution, banks are experiencing a fast-paced digital transformation. Lloyds Banking Group recently allocated a £3 billion strategic investment to the development of digital banking products and upskilling of its staff for the digital age. In addition, Deutsche Bank spends over $4 billion on technology every year while JP Morgan dedicates 16% of its total budget to digital (Williams et al., “Sustainable AI in Finance: Understanding the Promises & Perils”). AI technology is particularly well-adapted to financial services where data is abundant and the needs for structured problem solving are high. AI’s coming of age in the post-global financial crisis era furthermore coincides with the strong drive among banks to enhance their compliance efforts.
Automation is a most prominent feature of the adoption of AI by financial institutions. Client-facing chatbots or ‘virtual assistants’ are actively being developed by forward-looking banks to improve customer interactions and help employees solve complex problems. BNY Mellon took a substantial step in the AI direction by deploying over 220 robots that handle repetitive tasks such as processing data requests and correcting mistakes. This AI initiative enabled BNY Mellon to achieve an 88% improvement in overall processing time (Williams et al., “Sustainable AI in Finance: Understanding the Promises & Perils”). As an illustration, the time required for AI to settle a failed trade is a quarter of a second compared with 5-10 minutes if handled by a human being. Moreover, AI is being incorporated by banks to reshape their capital optimization, risk modelling and business management models. Calculations to forecast and classify necessary levels of regulatory capital have quickly become the target of AI algorithms and artificial neural networks. AI technology can also be used in internal risk model valuation to detect anomalous projections generated by stress testing models.
AI in Fighting FinCrime and Money Laundering
In addition to servicing, enhancing customer relations and facilitating regulatory reporting, the use of AI by financial institutions importantly concentrates on Financial Crime (FinCrime) detection and anti-money laundering (AML) activities. Money laundering is estimated to cost the global economy $2 trillion per year, as much as Italy’s entire GDP (Williams et al., “Sustainable AI in Finance: Understanding the Promises & Perils”). This sum grows with each year. Governments have stepped up their regulatory requirements and oversight in response, while regulators have handed out record fines to banks found guilty of AML breaches. Global banks, which are under closer scrutiny, have in turn experienced an annual increase up to 50% in compliance costs over recent years (Williams et al.,“Sustainable AI in Finance: Understanding the Promises & Perils”).
The central challenge for financial institutions when fighting FinCrime and money laundering is to create operational efficiency while actively monitoring the institution’s risk profile. This is where AI can be a highly operational tool. In a recent project undertaken by Ayasdi and HSBC for example, the two organizations developed an intelligent segmentation of customers to decrease the number of false positives by more than 20% while capturing every previously discovered suspicious activity report (Williams et al., “Sustainable AI in Finance: Understanding the Promises & Perils”). The project also identified hitherto undetected behavioral patterns of interest, thereby enhancing the bank’s risk profile. Similar approaches to those used for improving FinCrime detection can further be applied to credit scoring to speed up lending decisions and reduce credit risk.
Risks and Concerns Related to Using AI in Finance
Threats to Job Security and Financial Stability
The rapid growth of AI raises several questions regarding the mitigation of its potential risks and negative impacts. Such questions are notably related to job security. While driving job creation in certain sectors of the economy, AI is likely to cause job losses in other sectors. AI is already altering the very nature of jobs in cutting-edge industries as demand is rapidly adapting to target profiles with strong data science, digital and machine learning skills.
The implications of AI use also raise the question of financial stability. A recent report by the Financial Stability Board (FSB) highlights the lack of transparency and auditability of AI algorithms in trading poses macro-level risks (Financial Stability Board, “Artificial Intelligence and machine learning in financial services”). Accordingly, the heightened application of AI could result in new and unexpected interconnectedness between financial markets based on the use of previously unrelated data sources in the design of hedging and trading strategies. AI and machine learning may also create ‘black-boxes’ in decision-making as it becomes increasingly difficult for financial actors to grasp how trading and investment decisions are formulated by technology (Williams et al., “Sustainable AI in Finance: Understanding the Promises & Perils”). The communication mechanism used by AI tools may be opaque to humans, posing monitoring challenges for the operation of AI solutions.
Another concern relates to new sources of market concentration in financial services, most particularly regarding third-party relationships. A strong network effect and first-mover advantage may foster a ‘winner-takes-all’ dynamic, leading the UK’s Financial Conduct Authority (FCA) to reinforce supervisory efforts on third-party dependencies and supply chain risks in the banking industry (Williams et al., “Sustainable AI in Finance: Understanding the Promises & Perils”).
Transparency, Traceability and Trackability
Drawing insights from an unprecedented amount of data calls for increased human oversight and guidance. AI can only be as effective and beneficial as the supervisory systems put in place to monitor its development. Ensuring that the financial industry fully benefits from AI requires a clear definition of this technology’s allowed reach, notably as regards privacy and cybersecurity. The responsible and informed adoption of AI by the financial sector thus involves closer coordination across financial institutions and active role-taking by internal risk management and audit teams.
To comply with the upcoming more stringent AI regulations in the United States and the rest of the world, companies will need to integrate new tools and processes such as data protocols, system audits, AI monitoring, and awareness training. Google, Microsoft and BMW are among the key players currently developing formal AI policies with commitments to safety, transparency, fairness and privacy (Candelon et al., “AI Regulation Is Coming”). Companies such as the Federal Home Loan Mortgage Corporation have appointed chief ethics officers to oversee the introduction and enforcement of transparency, traceability and trackability measures, sometimes supporting them with ethics governance boards. Finally, the EU highlighted explainability in its white paper and AI regulation proposal as a core factor to promote trust in AI and thereby increase its adoption (Candelon et al., “AI Regulation Is Coming”).
Ethics Issue Spotting
Before unlocking the transformative abilities of AI, firms and financial institutions must understand that AI is not a standalone but transversal technology. AI’s benefits are closely linked with the development of alternative technologies and capacities such as blockchain, cloud and quantum computing (Deloitte, “Artificial intelligence in investment management”). It is essential for businesses that are considering adopting AI to reflect on how AI fits within their models and strategic objectives and consider potential risks. The successful wide-scale adoption of AI in finance importantly requires firms work with a diversified set of industry stakeholders and actively collaborate with associations and regulators (Deloitte, “Artificial intelligence in investment management”). Embracing partnerships can also be helpful to solve problems collectively, develop impactful sustainable solutions and foster the long-term development of best practices in responsible AI.
Initiatives such as the ‘Partnership on Artificial Intelligence’, founded in September 2016 by a group of large tech firms including Amazon, Facebook, Google, IBM and Microsoft, are actively promoting ethical, environmentally conscious and socially beneficial uses of AI (Financial Stability Board, “Artificial Intelligence and machine learning in financial services”). The Partnership, which now counts over 50% of non-profit organizations among its partners, has grown to become one of the largest groups of AI engineers working with non-experts to produce guidelines and best practices in key areas of AI.
Diversity
The AI research community has a key role to play in encouraging and supporting diversity and inclusion. A recent study by the World Economic Forum found that 78% of Linkedln users who self-identify as possessing AI skills are men (Fung, “This is why AI has a gender problem”). Research by Element.ai further shows that across three machine learning conferences held in 2017, 88% of the AI researchers who participated were men. Virtual assistants and conversation companions, in turn, are typically equipped with female voices and names while search and rescue robots like Hermes and Atlas are built in the male form (Fung, “This is why AI has a gender problem”). Siri, Alexa and Cortana were traditionally designed to feature female-sounding voices, although they have been updated since. The financial sector itself is not immune, for example, Natwest’s Cora and Bank of America’s Erica are two customer-facing robots with female names that provide day-to-day banking guidance and assistance.
Discrimination
An investigation by The Markup has moreover found that lenders using AI technology in loan applications are more likely to deny home and short-term loans to people of color (Cotton, “Regulators warn of discriminatory AI loan applications”). The Markup’s investigation revealed that 80% of Black applicants, 40% of Latino applicants and 70% of Native American applicants are more likely to be denied a loan compared to other applicants. Such results are relevant considering that 45% of the United States’ largest mortgage lenders offer online or app-based loans (Cotton,“Regulators warn of discriminatory AI loan applications”). As robots and voice assistants become increasingly more prevalent, it is important to be aware of and reactive to how technology depicts and processes characteristics such as gender, race, ethnicity and age, and how the composition of AI development teams may impact these portrayals.
According to Juniper Research there are over three billion voice assistants in use in the world (Chin & Robinson, “How AI bots and voice assistants reinforce gender bias”). The growing reliance on AI technology worldwide thus importantly calls for a robust commitment on the part of AI developers and tech companies alike to actively promote and ensure diversity and inclusion toward their users and among their teams, coupled with the establishment of clear standards and guidelines for the development of responsible, accountable and transparent but also respectful AI.
References
“Artificial intelligence: The next frontier for investment management firms”. Deloitte, 2019. https://www2.deloitte.com/content/dam/Deloitte/global/Documents/Financial-Services/fsi-artificial-intelligence-investment-mgmt.pdf
Candelon et al., “AI Regulation Is Coming”. Harvard Business Review, September–October 2021. https://hbr.org/2021/09/ai-regulation-is-coming
Chin, Caitlin, and Mishaela Robinson, “How AI bots and voice assistants reinforce gender bias”. Center for Technology Innovation, The Brookings Institution, November 2020. https://www.brookings.edu/research/how-ai-bots-and-voice-assistants-reinforce-gender-bias/
Cotton, Barney, “Regulators warn of discriminatory AI loan applications”. Business Leader, February 2022. https://www.businessleader.co.uk/regulators-warn-of-discriminatory-ai-loan-applications/
“DreamQuark: The Sustainability Imperative for Financial Services To Embrace Responsible AI”. The Fintech Times, July 2021. https://thefintechtimes.com/dreamquark-the-sustainability-imperative-for-financial-services-to-embrace-responsible-ai/
Financial Stability Board. “Artificial Intelligence and machine learning in financial services”. November 2017. https://www.fsb.org/2017/11/artificial-intelligence-and-machine-learning-in-financial-service/
Fung, Pascale, “This is why AI has a gender problem”. World Economic Forum, June 2019. https://www.weforum.org/agenda/2019/06/this-is-why-ai-has-a-gender-problem/
Gillham, Jonathan et al. “How AI can enable a sustainable future”. PWC UK, April 2020. https://www.researchgate.net/publication/340386931_How_AI_can_enable_a_Sustainable_Future
S&P Global, “How can AI help ESG investing?”. February 2020. https://www.spglobal.com/en/research-insights/articles/how-can-ai-help-esg-investing
Vinuesa, Ricardo et al. “The role of artificial intelligence in achieving the Sustainable Development Goals”. Nature Communications, January 2020. https://www.nature.com/articles/s41467-019-14108-y
Williams, Alan et al. “Sustainable AI in Finance: Understanding the Promises & Perils”. Parker Fitzgerald, May 2018. https://parker-fitzgerald.com/?news=sustainable-ai-in-finance-understanding-the-promises-and-perils*
Image Courtesy of Gerd Altmann